European Engineering Educators podcast: season 1 episode 4: Jan van de Veen from TUE discusses interdisciplinary…
European Engineering Educators podcast: season 1 episode 2:
Mia Stephens from JMP discusses statistical thinking with Natalie Wint and Neil Cooke
For our second episode we welcome Mia Stephens, an Advisory Product Manager with JMP (pronounced jump), a corporate partner of SEFI, and part of SAS Institute, an American multinational developer of analytics software. Mia is responsible for providing technical support for JMP academic users. Prior to joining SAS, she was an Adjunct Professor of Statistics at University of New Hampshire, a founding member of the North Haven Group and a trainer and consultant with the George Group. As well as being a co-author of four books and several papers, she has developed training materials, taught, and consulted within a variety of fields and industries.
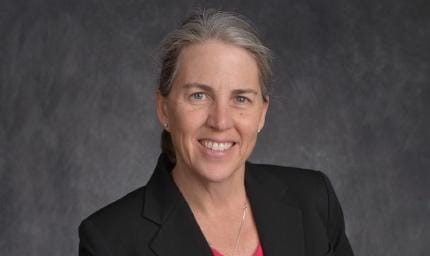
In this episode Mia explains that statistical thinking is key to understanding engineering processes and process variation, and thus process improvement. She explains that many academic programs don’t teach students to think statistically, and about the existence of a skills gap between what is taught and what industry needs. She introduces us to STIPS (Statistical Thinking for Industrial Problem Solving), a free online statistics course that introduces the statistical thinking process through 7 modules (statistical thinking and problem solving; exploratory data analysis; quality methods; decision making with data; correlation and regression; design of experiments; and predictive modeling and text mining.
Link to the podcast:
Background
Engineers are often defined as problem solvers who aim to make the world a better place by designing, creating, and improving products and systems. However, to improve something, we must first understand it. This involves collecting, managing, and analyzing data so that we can make accurate and reliable predictions about the long-term behavior of the products we design.
“Measurement is the first step that leads to control and eventually to improvement. If you can’t measure something, you can’t understand it. If you can’t understand it, you can’t control it. If you can’t control it, you can’t improve it” (H. James Harrington, Quality Lead, IBM)
However, data is only useful if we understand where it comes from and if we analyze it correctly. The rapid increase in the availability of data (IDC, 2021) has thus created a growing need to reflect on and critique the use of data.
In the second episode of the European Engineering Educators Podcast, we welcome Mia Stephens, an Advisory Product Manager with JMP, a corporate partner of SEFI, and part of SAS Institute, an American multinational developer of analytics software. Mia’s time teaching and consulting in both academia and industry enabled her to bring a range of perspectives to her role as Lead Developer of the STIPS (Statistical Thinking for Industrial Problem Solving) online statistics course. It is perhaps this which has helped bridge the gap between how statistical thinking is taught, and how it is applied within the field.
The remainder of this article will summarise the key discussion points. you may wish to reflect on after listening to the episode.
Statistical thinking is about understanding that all engineering work (including design) occurs in a system of processes
Within each process and each process step, there is variation. To make improvements or optimize a process we must understand this variation. Statistical thinking and making decisions with data thus rely on quantification and interpretation of variation.
There is a disconnect between how statistical thinking is taught and how it is applied within the field.
To be effective, students need to be taught statistics in a practical and applied manner. Currently, it is typically taught in a very theoretical way and is often based on calculus. However, students should instead gain experience in visualizing and interacting with data.
The heavy workload associated with engineering programs can also lead to a lack of appreciation for statistical thinking from both academics and students. Students often need practical, first-hand experience of encountering variation within industry before understanding the power of statistics.
Statistics as detective
Mia contends that it is not always necessary to rely on ‘heavy’ statistical tools such as hypothesis testing, regression analysis and statistics modeling, to gain insights into processes and detect failures. Instead, we can take a more practical, process and problem-orientated approach to problem-solving and make use of exploratory data tools. Exploring and investigating our data can allow us to gain insights that can lead to design of experiments or to us identifying which areas of a process to look at more closely. For example, we can visualise data using simple tools such as run charts, box plots, and histograms.
Courses should therefore be as applied as possible, mirroring how statistics is taught and used in industry. Mia highlights three core concepts that should be taught including: picking the right tool, asking questions; and interpreting what the results are telling you. She also mentions three different quality tools which are particularly important within industrial settings. Firstly, statistical process control (understanding behavior in your process over time), measurement system analysis (the degree to which a measurement system is capable of producing reliable results), and capability analysis (the degree to which a process is capable of meeting specifications).
Design of experiments (DoE) as most powerful tool in any engineering toolkit
Statistically designed experiments involve creating a map of a process wherein there are a series of interconnected steps with associated parameters, inputs, and outputs. Design of experiments can then be used to understand how each step (and its associated parameters) influences the output of the entire process with respect to one characteristic. This understanding of cause and effect can facilitate optimization. Different types of experiments can be used depending on what you want to learn. For example, a screening experiment is used to understand which factors are important in influencing the output process.
STIPS (Statistical Thinking for Industrial Problem Solving) as a tool to help students bridge the gap between education and industrial
The self-paced, online course is considered to be for anyone and everyone and introduces the statistical thinking process through 7 modules (statistical thinking and problem-solving; exploratory data analysis; quality methods; decision making with data; correlation and regression; design of experiments; and predictive modeling and text mining). Each module includes short instructional videos, demonstrations, practice exercises and quizzes.
Further information about the course can be found here.
https://www.jmp.com/en_no/online-statistics-course.html
Visual Six Sigma: Making Data Analysis Lean | JMP
The podcast episode is here:
https://shows.acast.com/european-engineering-educators/1-roland-tormey-from-epfl-discusses-ethics
References
ASQ Statistics Division, Glossary and Tables for Statistical Quality Control, Fourth Edition, (Milwaukee, WI: ASQ Quality Press, 2004).
Harrington, H. James (1987), Poor-Quality Cost, American Society for Quality, ISBN 978-0-8247-7743-2